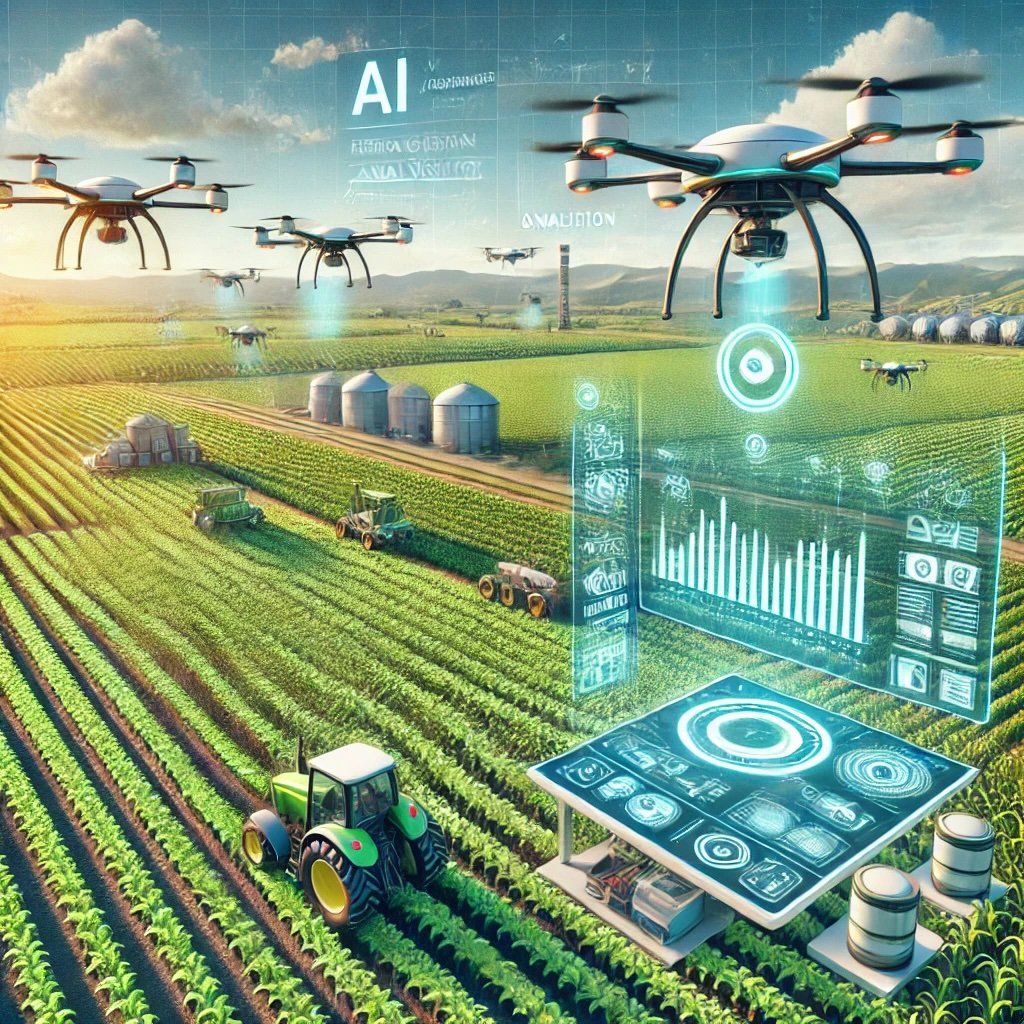
Boosting Agricultural Productivity with AI-Powered Image Analysis
The integration of AI into agriculture heralds a new era of farming, characterized by precision, sustainability and economic viability. By embracing AI, farmers can significantly enhance crop health monitoring, optimize resource use and adapt quickly to market and environmental changes. This isn't just about staying competitive; it's about transforming agriculture to meet the demands of a growing, more environmentally conscious population. Now is the moment to explore AI solutions tailored to your farm's unique needs, ensuring that your practices are not only productive but also sustainable for the future.
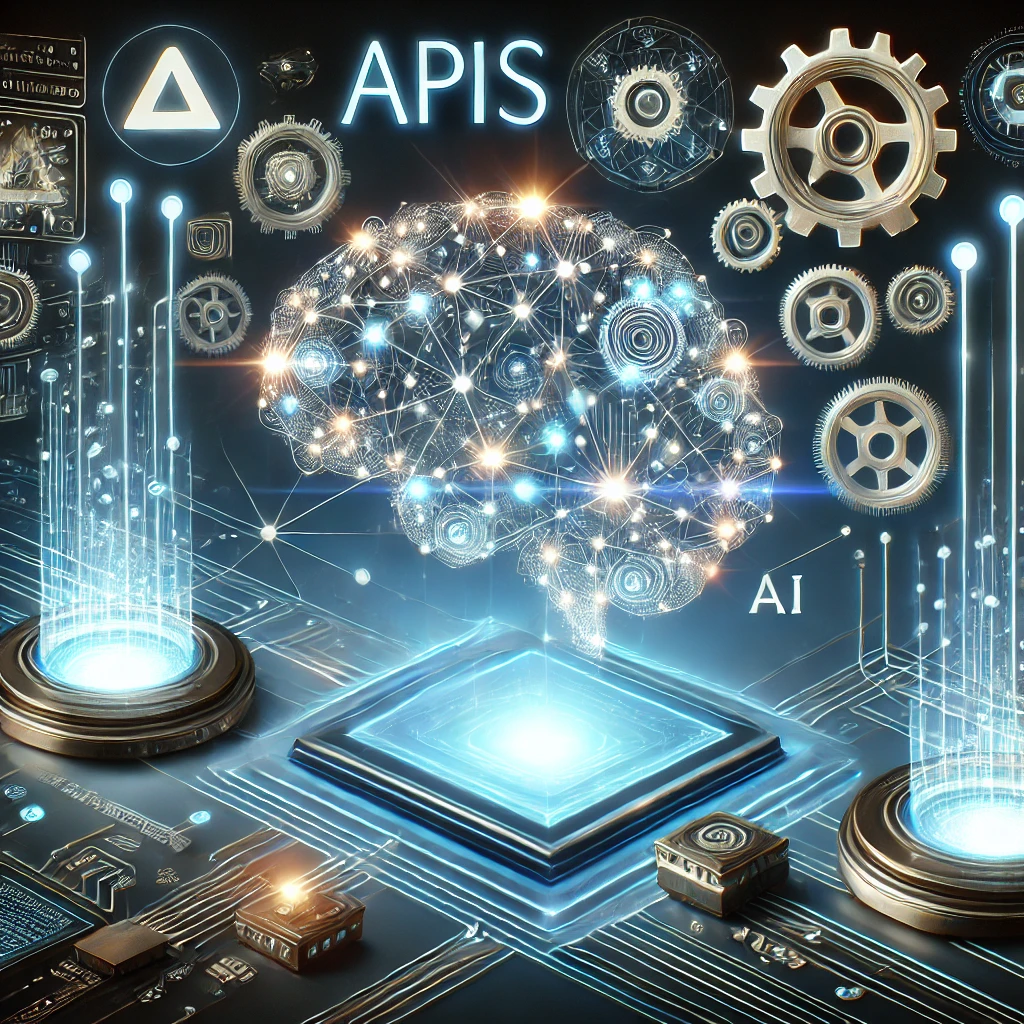
APIs for AI: The Ideal Fusion of Tools and Technology
Artificial intelligence is transforming industries, but integrating AI from scratch can be costly and complex. AI-powered APIs bridge this gap, offering businesses ready-to-use AI capabilities that enhance efficiency, automate tasks and drive innovation. From OCR for document automation to facial recognition for security, AI APIs make advanced technology accessible without requiring deep machine learning expertise.
In this blog post, we explore how AI APIs streamline business processes, improve scalability and future-proof operations. We also discuss key considerations for selecting the right AI API, the benefits of custom AI solutions and emerging trends like real-time AI processing and multimodal AI. Whether you're looking for quick AI integration or long-term strategic AI investment, APIs provide a flexible, scalable path to success in an AI-driven world.
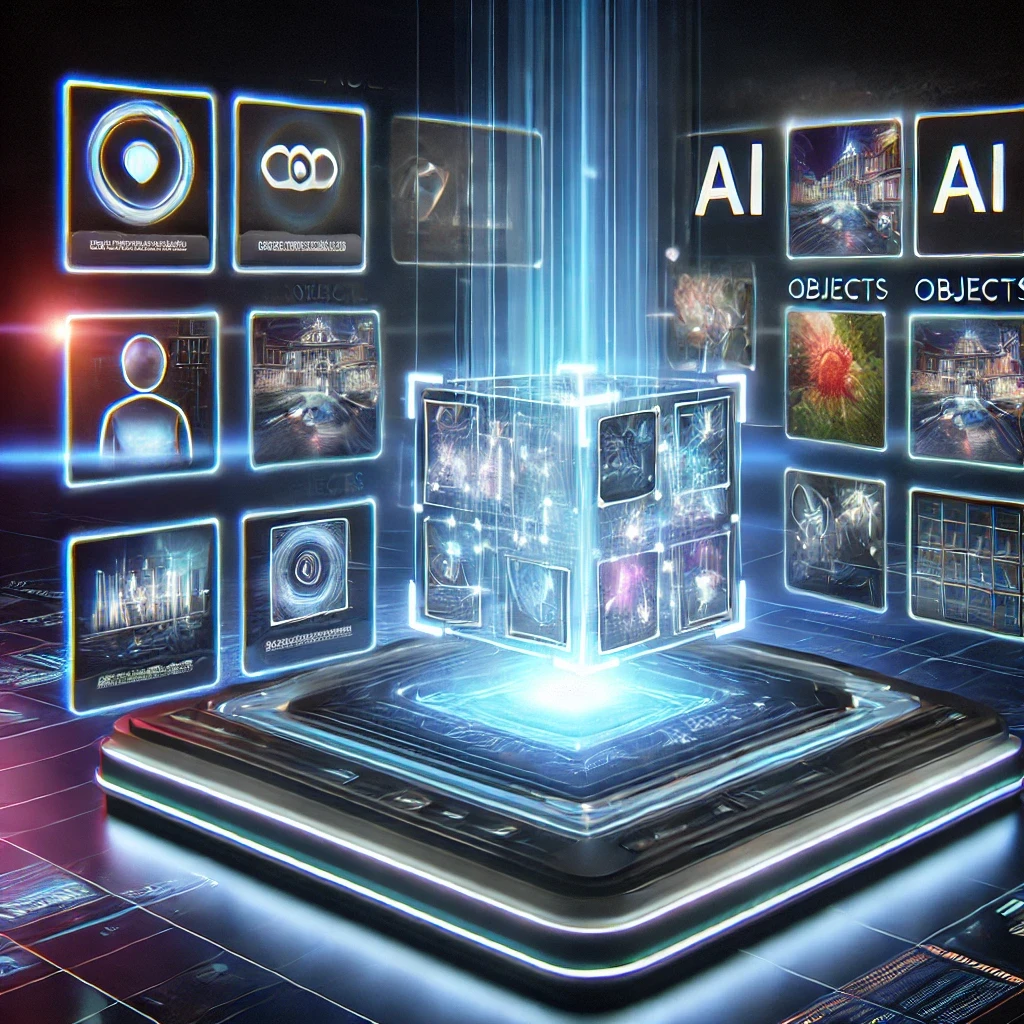
Smart Image Labeling: Unlocking the Full Potential of Visual Data
In today’s digital world, businesses generate and manage massive amounts of visual data. Whether it's e-commerce product catalogs, surveillance footage or industrial quality control images, efficiently organizing and analyzing this data is crucial. Smart image labeling, powered by AI, is transforming how industries handle visual information — automating categorization, improving searchability and uncovering actionable insights.
From object detection and OCR to contextual labeling and edge AI, modern image labeling goes beyond simple tagging. Businesses that embrace these technologies can reduce operational costs, improve decision-making and gain a competitive edge. By leveraging cloud-based APIs or custom AI solutions, companies can scale their image processing capabilities with ease.
As industries evolve, the need for intelligent, automated image labeling will only grow. Now is the time to explore AI-powered solutions that unlock the full potential of visual data — turning raw images into valuable assets that drive efficiency, innovation and long-term success.
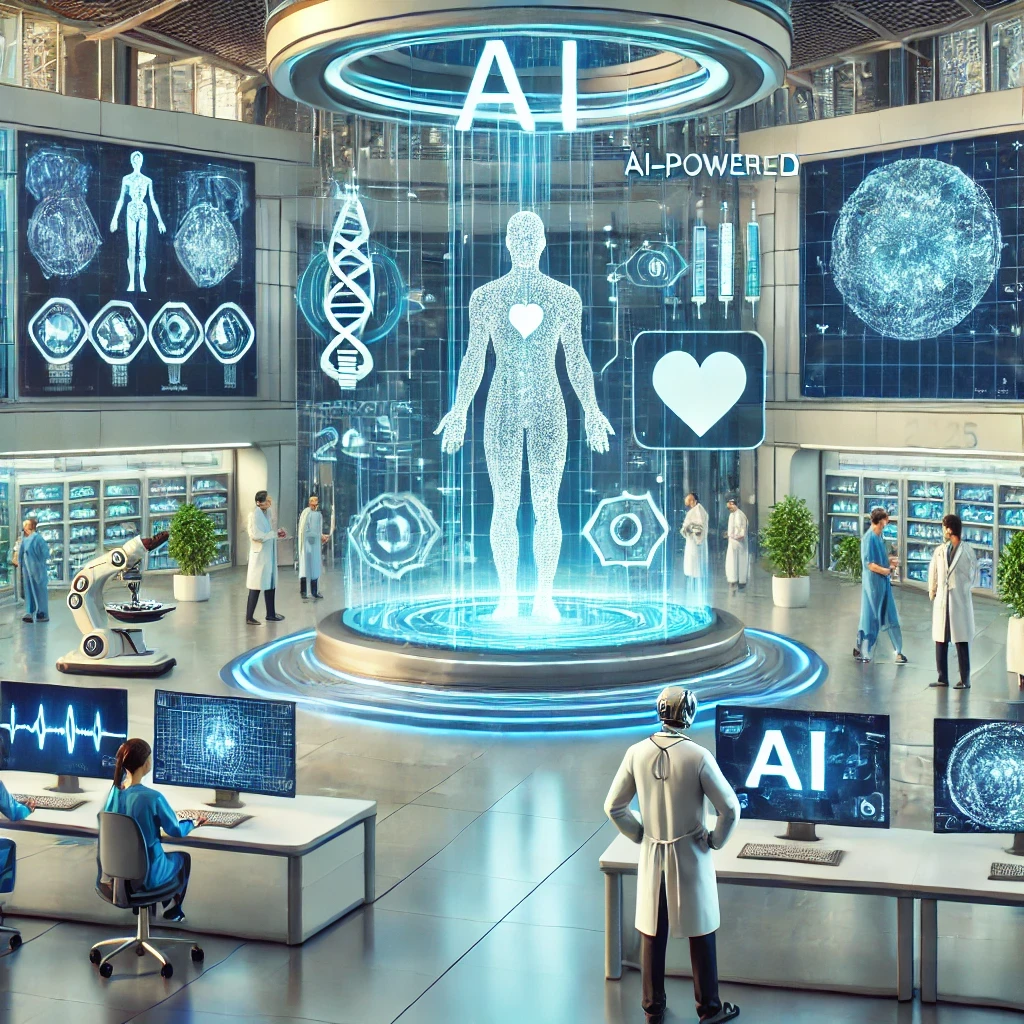
Top 10 AI Trends Shaping the Healthcare and Medical Industry in 2025
Artificial intelligence is reshaping the healthcare industry, revolutionizing diagnostics, treatment and hospital management. In 2025, AI-driven innovations are set to enhance medical imaging accuracy, enable personalized treatments, streamline telehealth services and optimize hospital workflows. From AI-assisted surgery to real-time patient monitoring, these advancements promise faster, more efficient and more accessible healthcare solutions. However, challenges such as data privacy, regulatory compliance and implementation costs must be carefully navigated. As AI continues to evolve, healthcare providers must embrace strategic adoption, ensuring scalable and ethical integration. The future of medicine is AI-powered, transforming patient care into a smarter, data-driven experience.
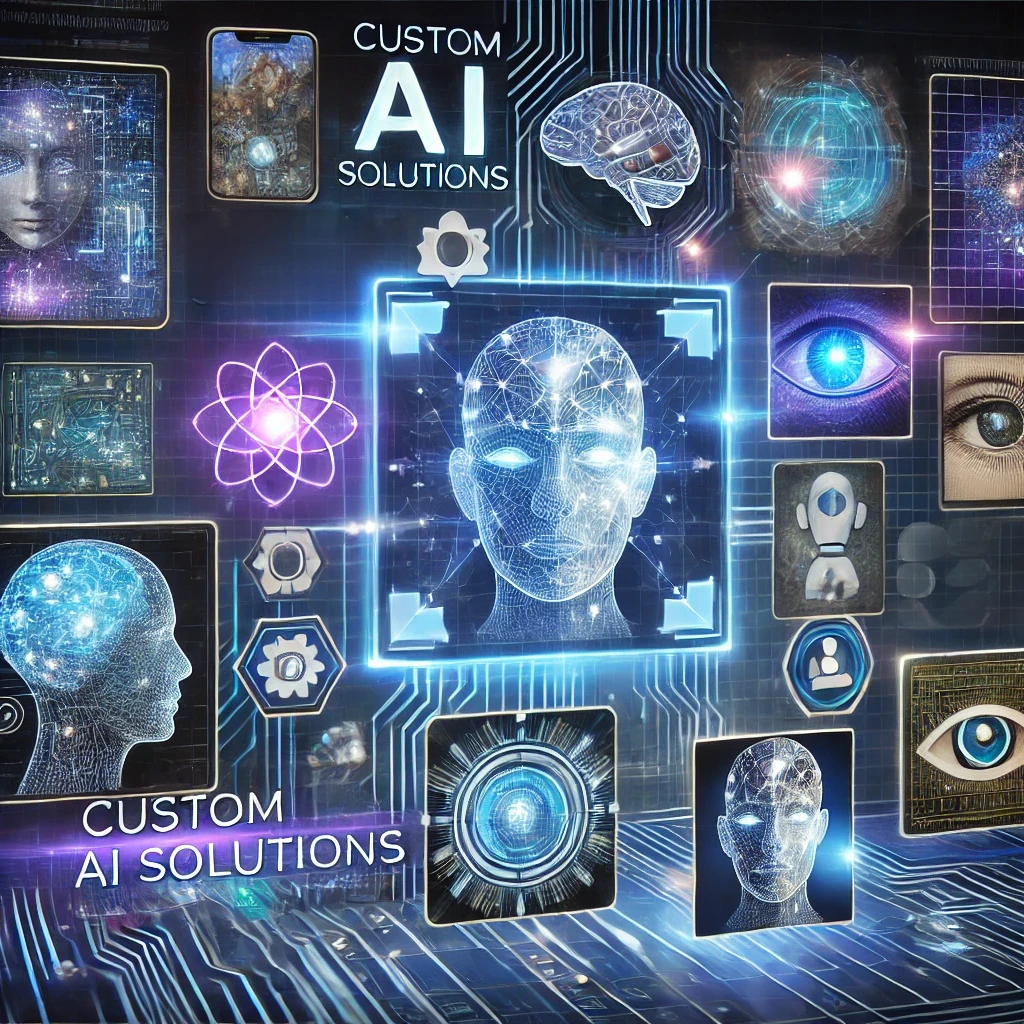
The Ultimate Guide to Custom AI Solutions for Image Processing
AI-powered image processing is transforming industries by automating workflows, improving accuracy and uncovering new business opportunities. While off-the-shelf AI solutions provide quick deployment, they often fall short when handling specialized tasks. Custom AI solutions offer greater flexibility, higher precision and long-term cost savings by adapting to unique business needs.
This guide explores the key benefits of tailored AI strategies, from boosting operational efficiency to maximizing return on investment. Businesses looking to integrate AI into their image processing workflows will learn how to assess their needs, develop a strategic implementation plan and future-proof their AI investments. As advancements in augmented reality, edge AI and robotics continue to shape the industry, organizations that embrace adaptable AI solutions will stay ahead of the competition.
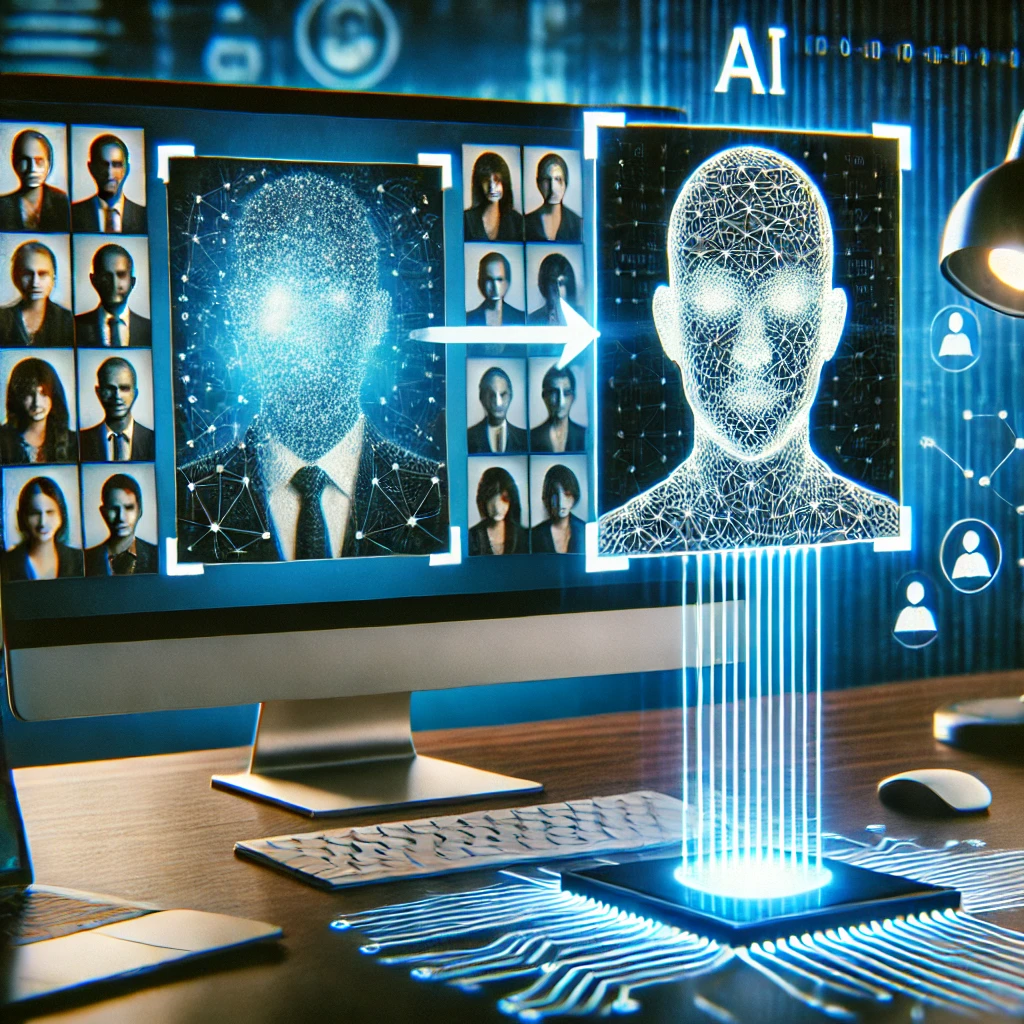
AI-Powered Image Anonymization: Ensuring Privacy and Data Protection
In an era where digital privacy is under constant scrutiny, AI-powered image anonymization has become essential for businesses handling sensitive visual data. Whether it's protecting patient identities in healthcare, ensuring compliance with privacy laws or securing personal information in surveillance footage, AI-driven solutions provide an efficient, scalable and automated way to safeguard images and videos.
This blog post explores the key technologies behind AI anonymization, including face blurring, object detection and deep learning models designed to obscure personally identifiable information. We also examine real-world applications across industries such as security, e-commerce, automotive and media, showcasing how AI anonymization enhances privacy without compromising image usability.
As AI-based data security evolves, future trends point toward real-time video anonymization, multi-object detection and advanced compliance tools that help businesses navigate increasingly strict privacy regulations. Organizations that integrate AI-powered anonymization into their workflows now will gain a competitive edge in compliance, security and customer trust. Whether leveraging pre-built APIs or investing in custom AI solutions, businesses must act proactively to ensure privacy protection in an increasingly digital world.
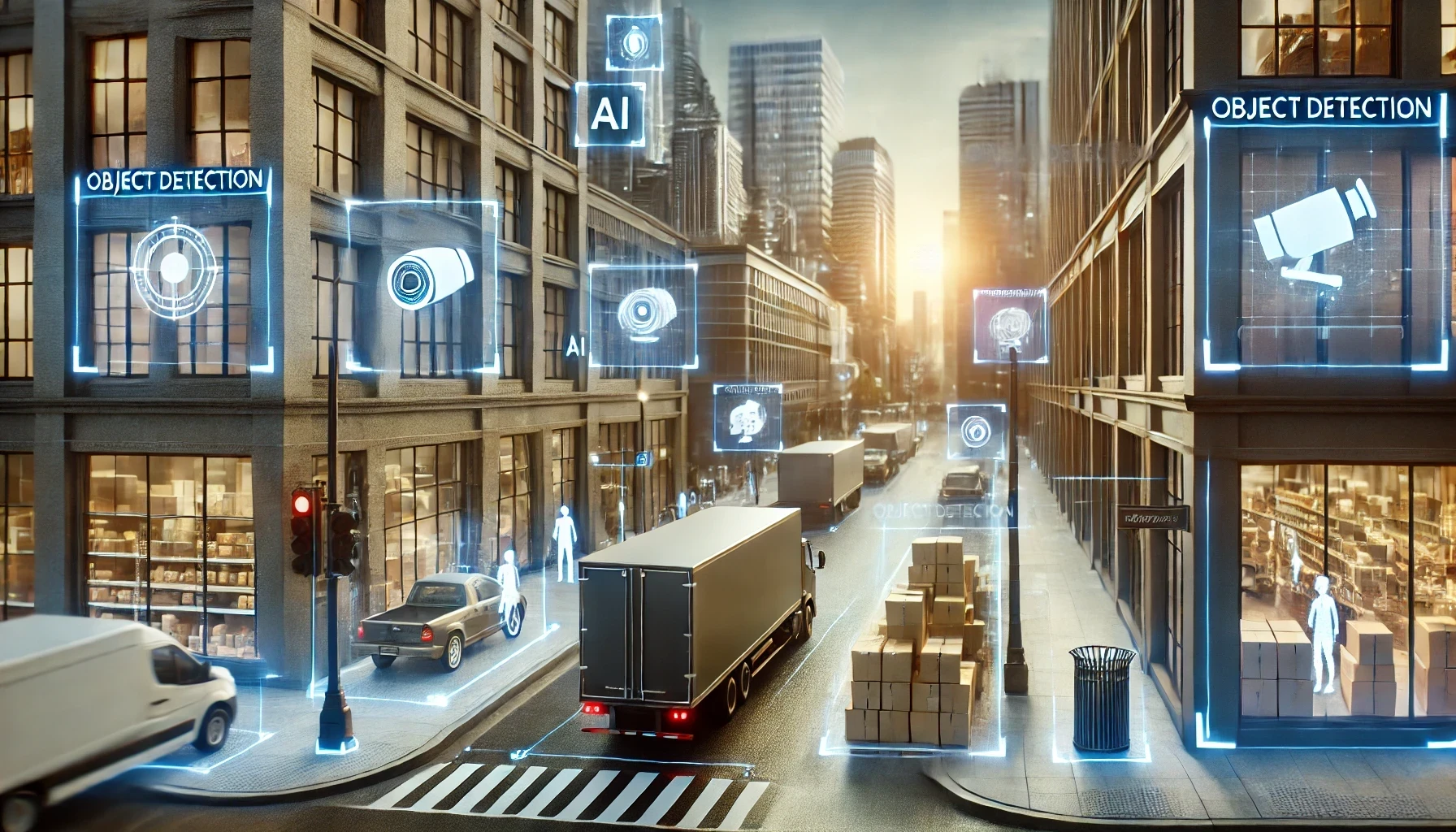
Object Detection APIs: Top Industry Applications & Benefits
In today’s data-driven world, businesses across industries are turning to AI-powered object detection APIs to automate image analysis, enhance efficiency and gain deeper insights. From e-commerce product tagging and manufacturing quality control to real-time security monitoring and personalized customer experiences, object detection is revolutionizing workflows and reducing reliance on manual processes.
This article explores the top industry applications of object detection APIs, highlighting their key benefits, including cost savings, scalability and competitive insights. Whether leveraging off-the-shelf solutions or investing in custom AI models, businesses can unlock new opportunities and future-proof their operations. As automation becomes essential for staying ahead, now is the time to explore how object detection can streamline processes, improve accuracy and drive innovation.
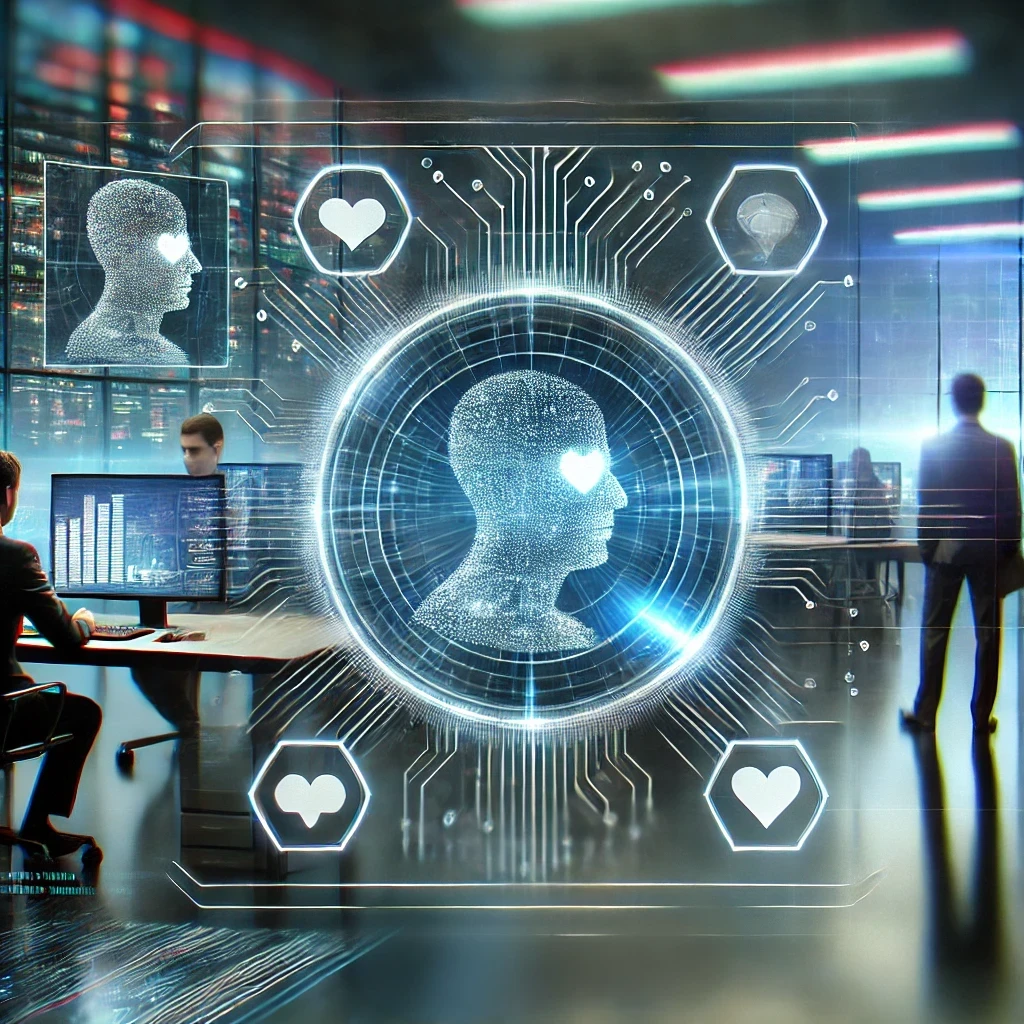
AI NSFW API: Top 5 Powerful Business Applications
In today’s digital landscape, businesses face an increasing challenge in moderating user-generated content while maintaining brand safety, user trust and compliance with evolving regulations. AI-powered NSFW detection has emerged as a game-changing solution, enabling platforms to automate content moderation, enhance efficiency and create a safer online experience.
This blog post explores the top five business applications of AI-driven NSFW detection, from e-commerce and social media platforms to digital advertising, video streaming and corporate compliance. It also delves into best practices, integration challenges and the future of AI-powered content moderation, offering valuable insights for companies looking to optimize their moderation workflows and future-proof their digital ecosystems.
Whether you're seeking off-the-shelf AI moderation tools or custom-built solutions, understanding how AI can improve accuracy, reduce operational costs and protect brand integrity is essential. Dive into this guide to discover how businesses are leveraging AI-powered NSFW detection to stay ahead in the evolving content moderation landscape.
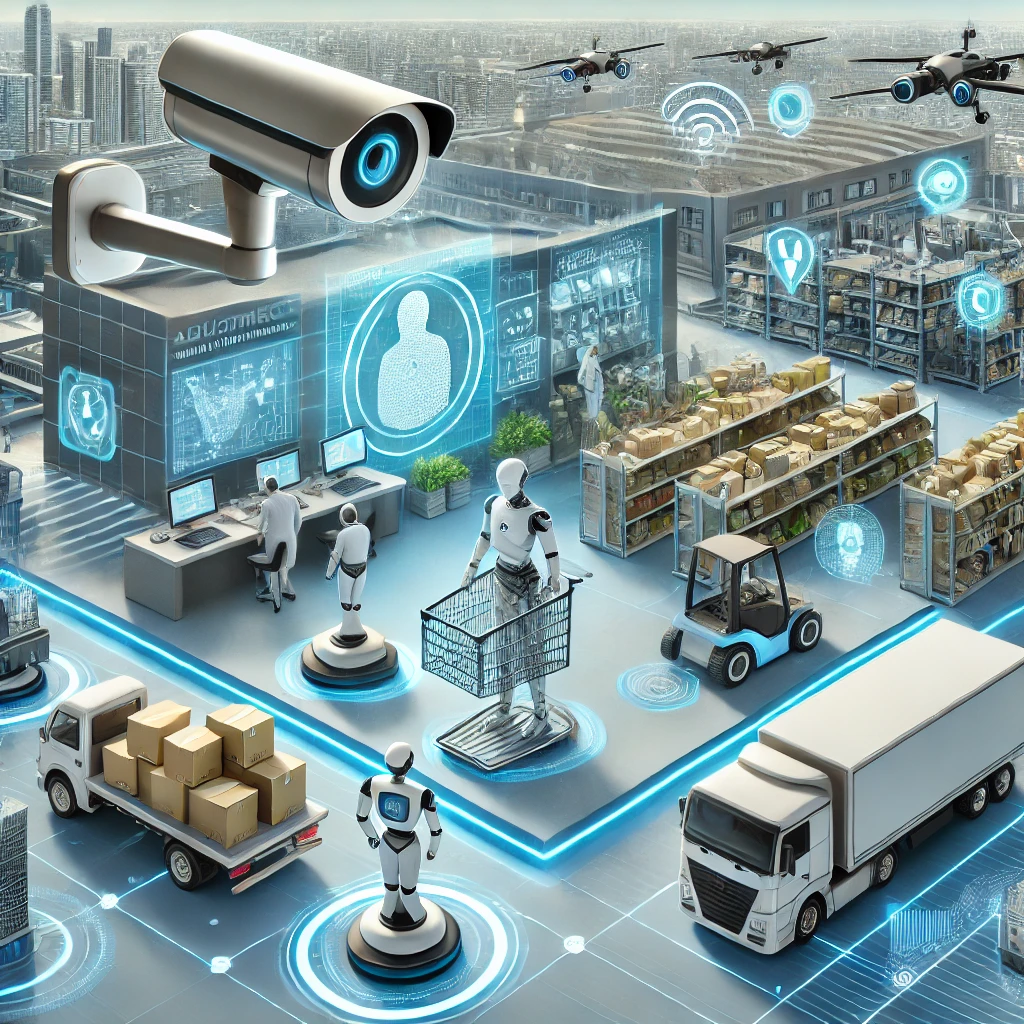
AI-Powered Object Detection API: Best Business Applications in 2025
As businesses increasingly rely on automation and data-driven decision-making, AI-powered object detection is emerging as a game-changing technology across industries. From real-time inventory tracking in retail to automated defect detection in manufacturing and AI-driven security systems, object detection APIs are streamlining operations, enhancing accuracy and unlocking new opportunities.
In this post, we explore the most impactful business applications of AI object detection in 2025, highlighting how companies can leverage off-the-shelf APIs for rapid deployment or invest in custom AI solutions for long-term competitive advantage. As AI continues to evolve, businesses that embrace this technology early will be positioned to lead in an increasingly digital economy.
Whether you're looking for a fast, scalable AI API or considering a tailored AI-powered solution, understanding the right integration strategy is key to maximizing ROI. Let's dive into how AI object detection is transforming industries and shaping the future of automation.
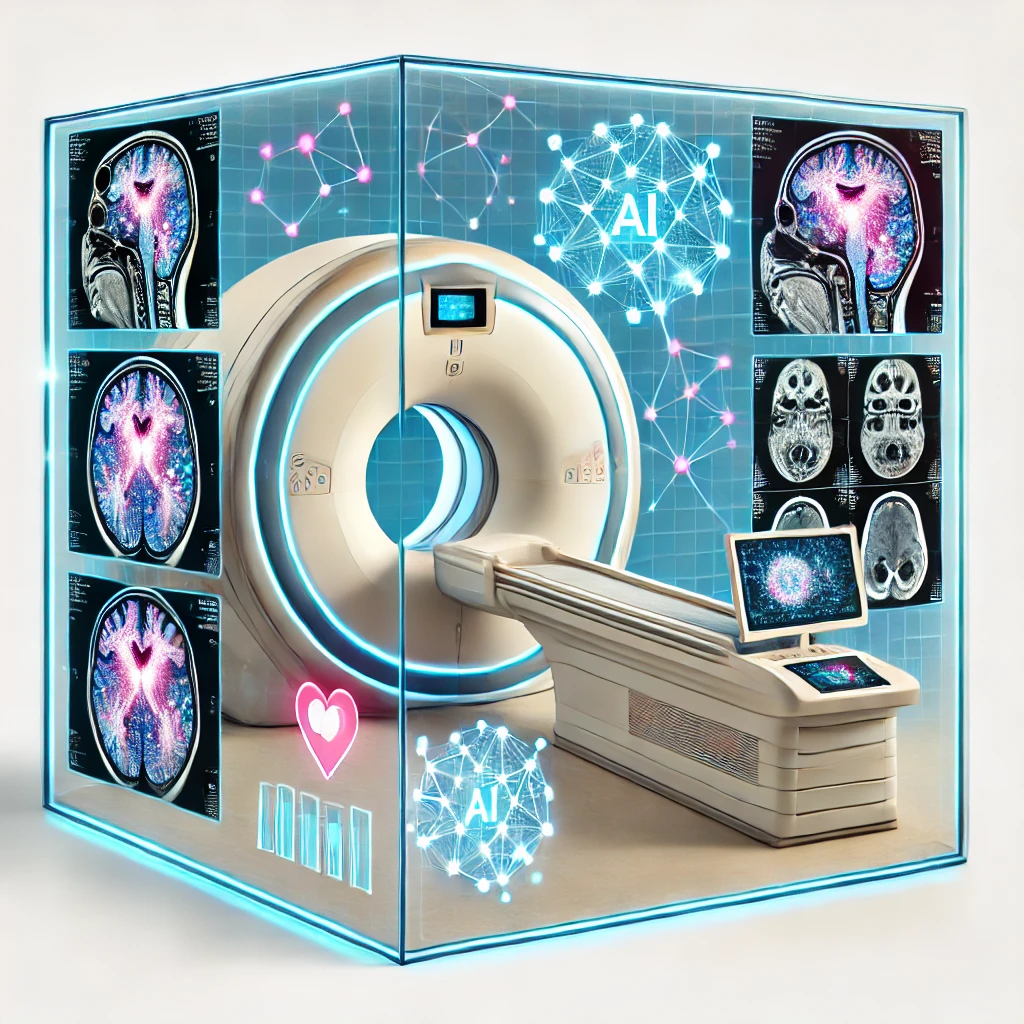
Medical Imaging: Deep Learning for Early Diagnosis
Deep learning is revolutionizing medical imaging, driving faster, more accurate diagnoses and paving the way for personalized medicine. By integrating advanced algorithms with medical workflows, healthcare providers can detect diseases earlier, improve patient outcomes and reduce costs. From real-time analysis and wearable integration to predictive analytics, this transformative technology offers endless possibilities for shaping the future of healthcare. Discover how tailored AI solutions are unlocking new potential in medical imaging and empowering healthcare professionals to deliver smarter, more efficient care.
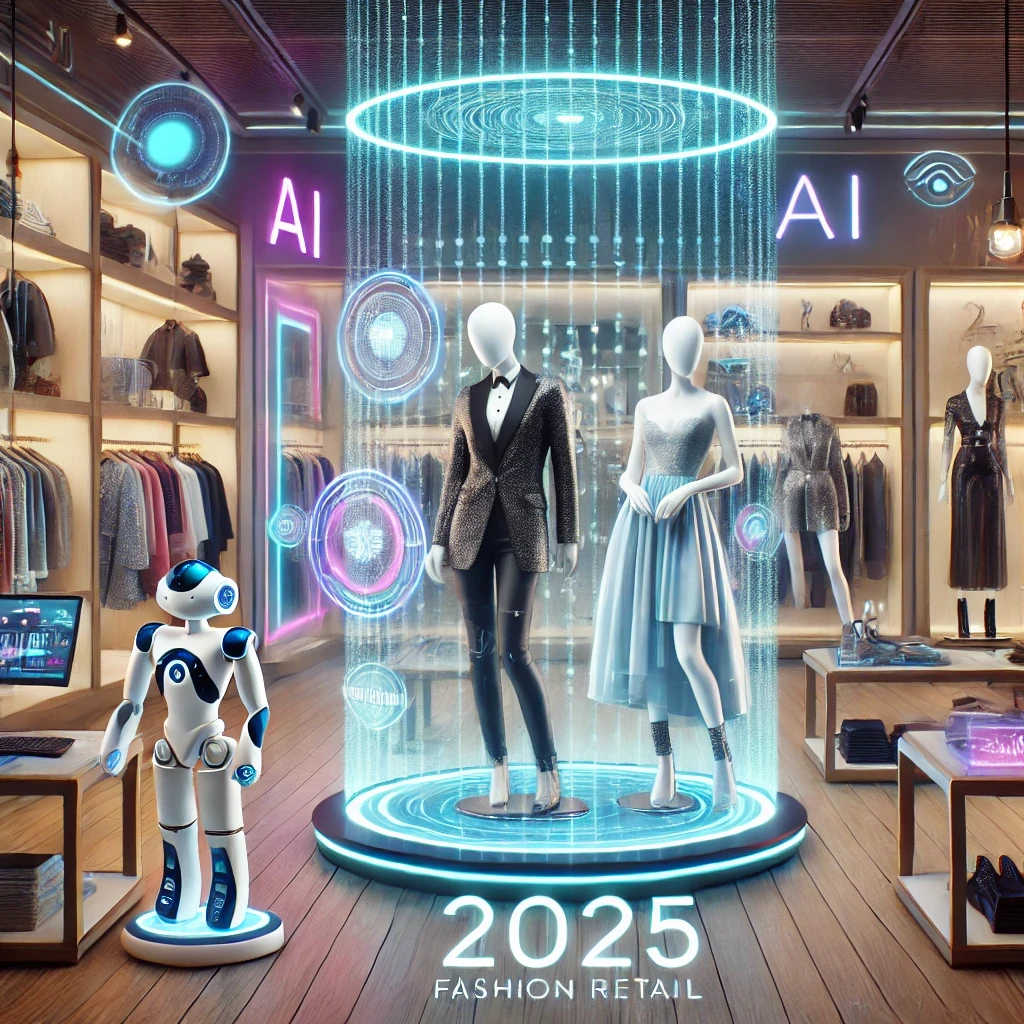
Top AI Trends for the Fashion Industry in 2025
The fashion industry is undergoing a digital transformation, with AI leading the charge in reshaping how brands design, produce and sell their products. From virtual try-ons and hyper-personalized shopping experiences to smarter inventory management and sustainable practices, AI-powered technologies are driving new opportunities for growth and efficiency. In this article, we explore the top AI trends that will define the fashion landscape in 2025, revealing how tools like visual search, augmented reality and custom AI solutions are revolutionizing customer experiences and supply chains. Discover how fashion brands can embrace AI to stay competitive and build a more ethical, innovative future.
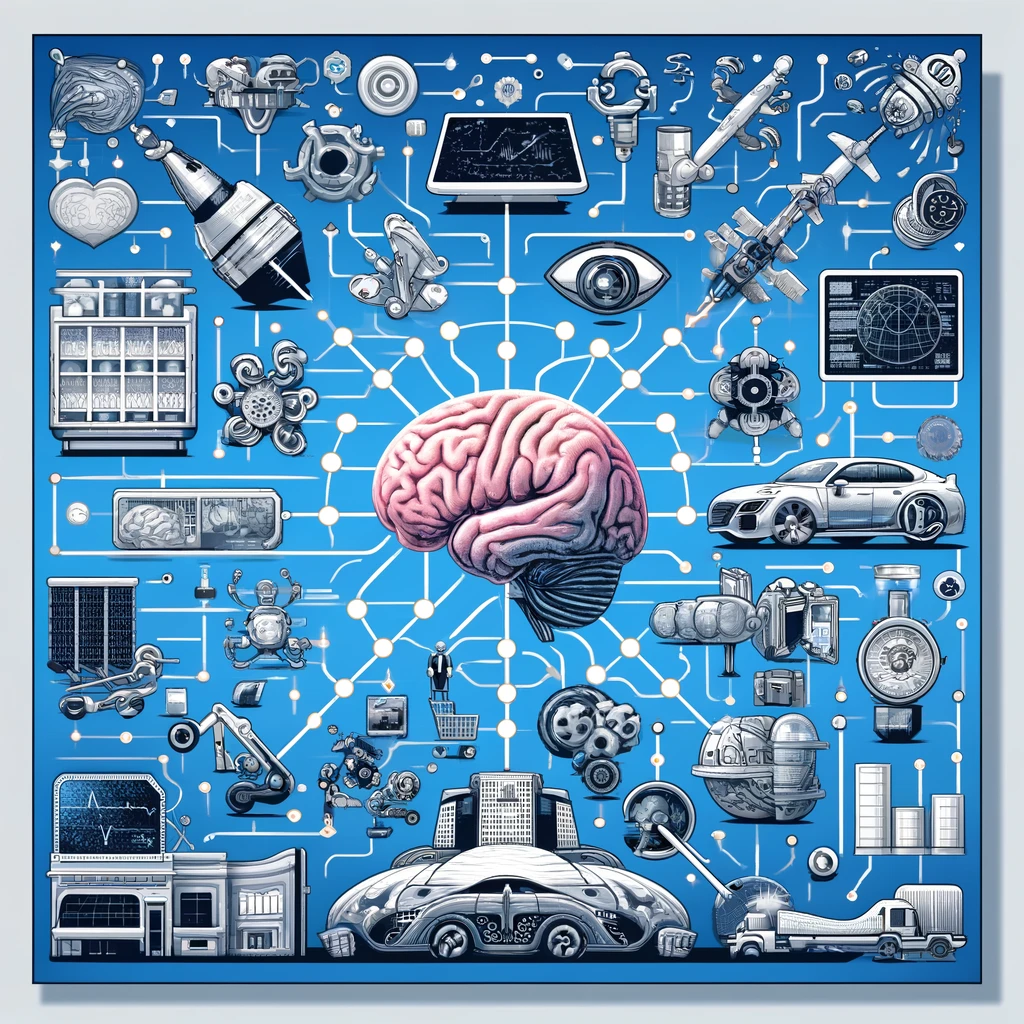
How Convolutional Neural Networks Transformed Industries Over the Past 10 Years
Over the last decade, Convolutional Neural Networks (CNNs) have revolutionized industries by transforming how businesses process visual data. From automating product recognition in retail to enhancing medical diagnostics in healthcare, CNNs have become the driving force behind modern image processing solutions. This blog post explores key breakthroughs in CNN technology, real-world applications across sectors and emerging trends like real-time edge processing and multimodal AI systems. As industries continue to evolve, adopting AI-powered image processing strategies is becoming essential for businesses to stay competitive, streamline operations and unlock new opportunities for growth.
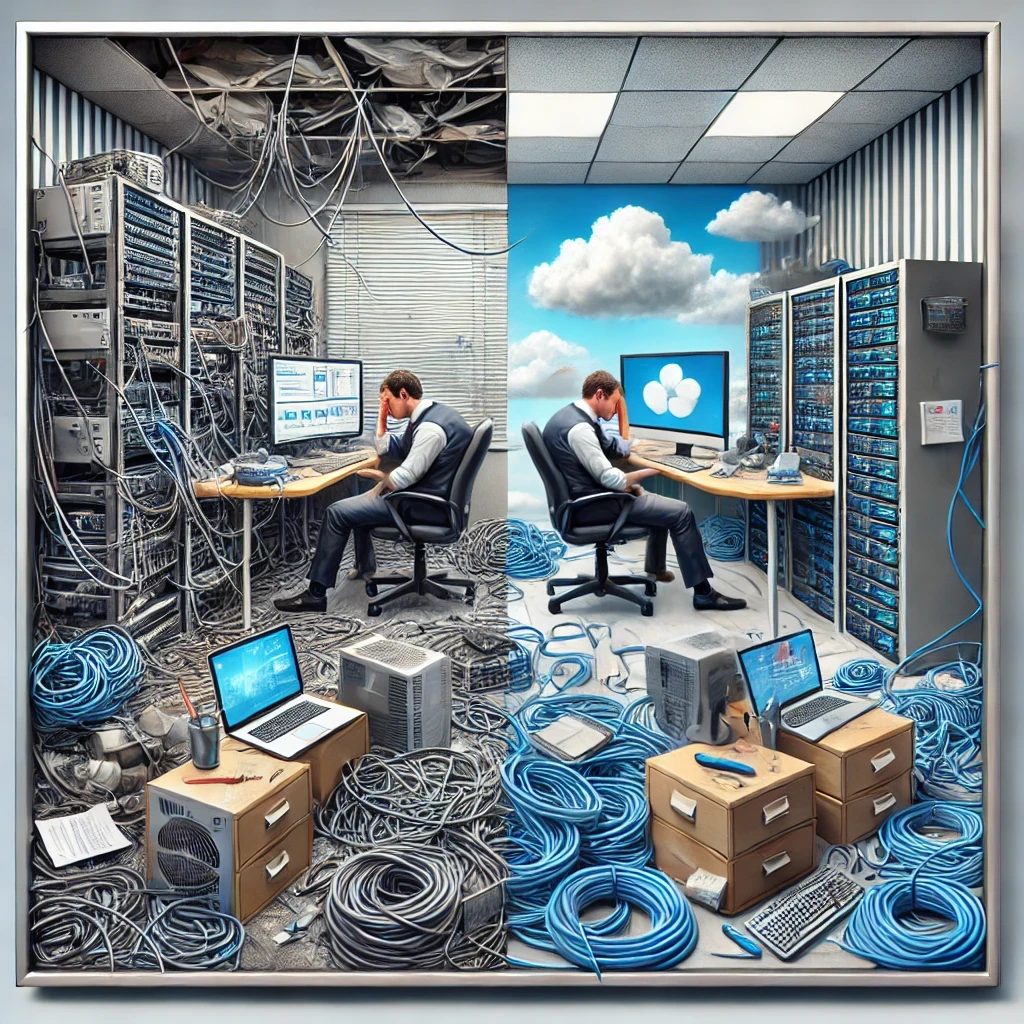
Key MLOps Challenges and Strategies for Small Businesses in Deep Learning
For small businesses adopting deep learning, managing AI models at scale can be a daunting task. Challenges like data management, costly training pipelines, deployment complexities and model drift can hinder success if not addressed properly. MLOps offers a solution by providing a structured framework to streamline AI workflows, improve model performance and reduce costs.
By leveraging cloud-based APIs, automated pipelines and continuous monitoring, small businesses can ensure their AI systems remain reliable and adaptable to real-world conditions. The future of MLOps will bring even more accessible tools, empowering small businesses to compete with larger players and innovate faster. Scaling AI isn't just for big enterprises — smart MLOps strategies can make it cost-effective and achievable for small businesses too.
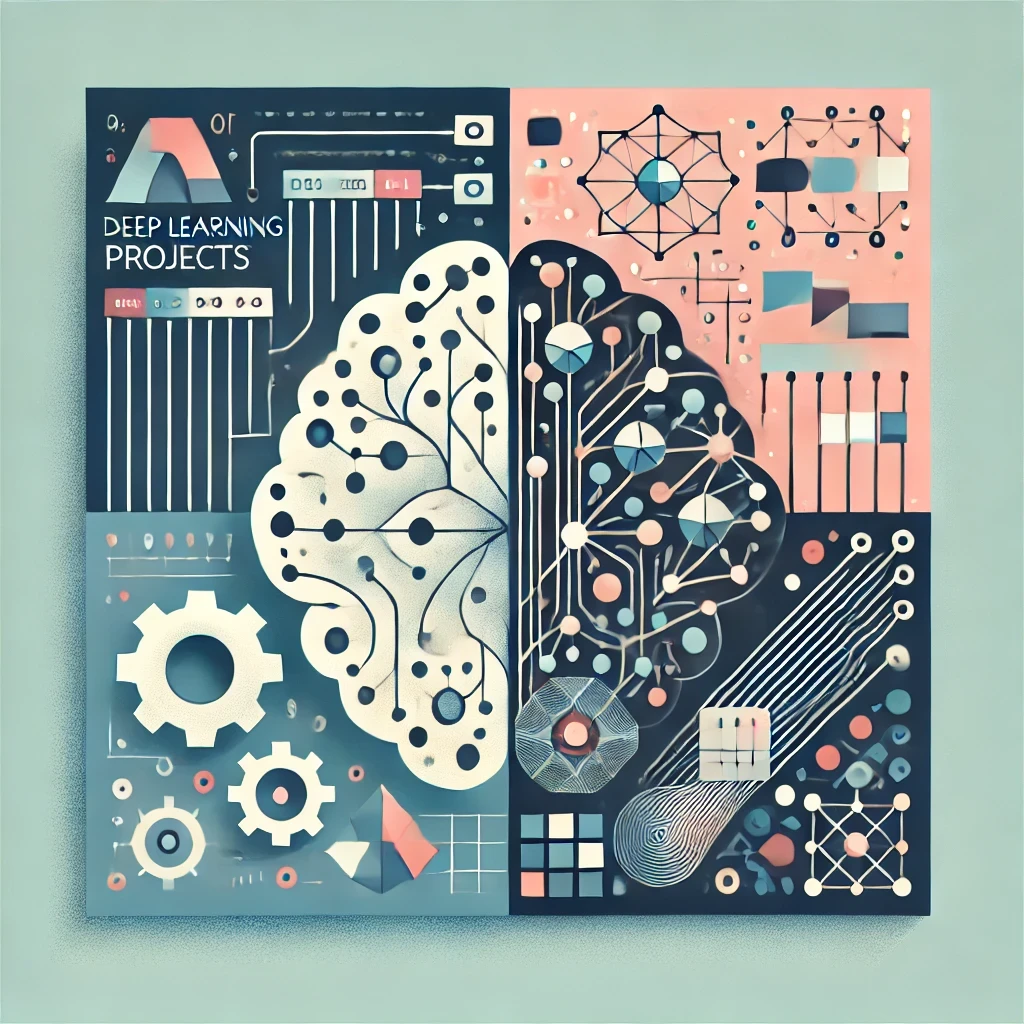
How Deep Learning Projects Differ from Traditional Software Development
Deep learning is revolutionizing how businesses approach software development. Unlike traditional rule-based systems, deep learning adapts and evolves through data-driven learning, enabling unparalleled scalability, innovation and problem-solving capabilities. In this blog, we explore the key differences between traditional software development and deep learning projects, the unique tools and workflows required and the benefits of embracing this transformative technology. Learn how API4AI empowers businesses with tailored AI solutions and scalable cloud AI APIs to meet their specific needs and prepare for a dynamic, future-ready world.
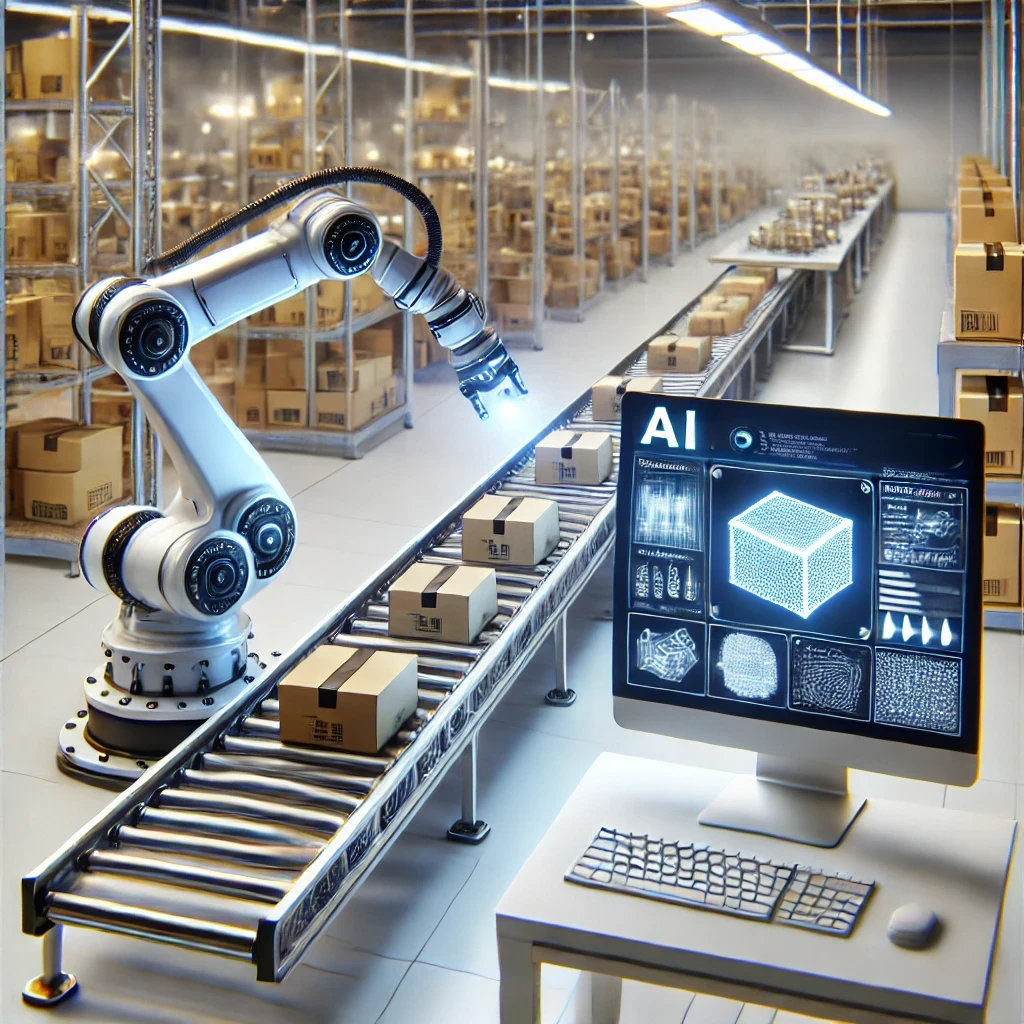
Quality Control 2.0: Detect Defects Faster with Deep Learning Models
Quality control is entering a new era with the power of deep learning. Traditional inspection methods, limited by human error and inefficiency, are being replaced by AI-driven manufacturing solutions that deliver unparalleled speed, precision and scalability. From detecting micro-defects in electronics to automating label inspections in pharmaceuticals, defect detection automation is transforming industries. Explore how deep learning revolutionizes quality control, its applications across sectors and why adopting AI-powered solutions is key to staying competitive in today’s fast-paced manufacturing world. The future of quality control is here — are you ready to embrace it?
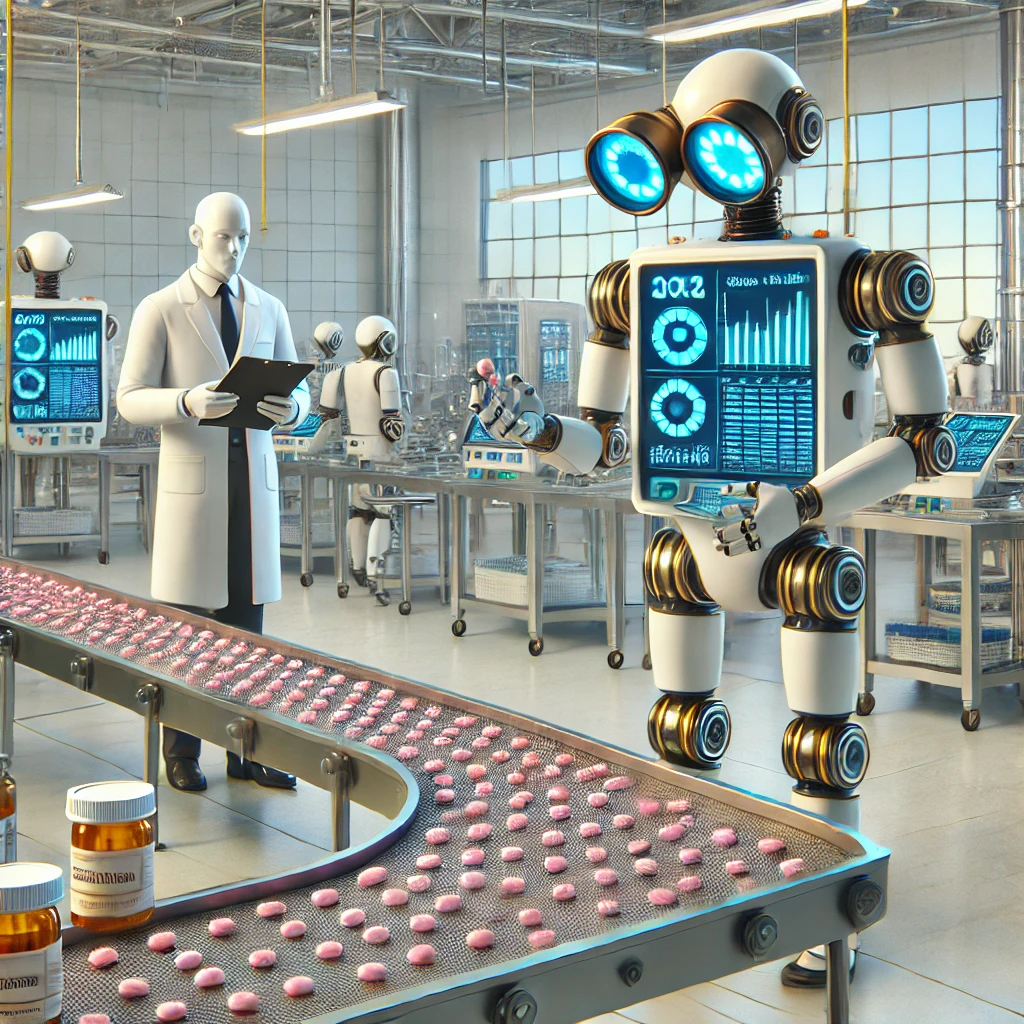
Custom AI Solutions for Quality Control in Pharmaceutical Manufacturing
In the pharmaceutical industry, quality control is paramount to ensuring patient safety and regulatory compliance. Traditional methods, while effective, often struggle with human limitations, scalability challenges and high operational costs. Enter custom AI solutions — tailored technologies that enhance precision, automate inspections and streamline compliance efforts. From detecting microscopic defects in tablets to verifying critical packaging details, AI-powered tools are transforming how manufacturers maintain quality at every step of production. By leveraging advanced technologies like image recognition, OCR and deep learning, pharmaceutical companies can achieve unparalleled efficiency and reliability. Ready to explore the future of smarter, safer manufacturing? Discover how custom AI solutions can meet your unique challenges today.
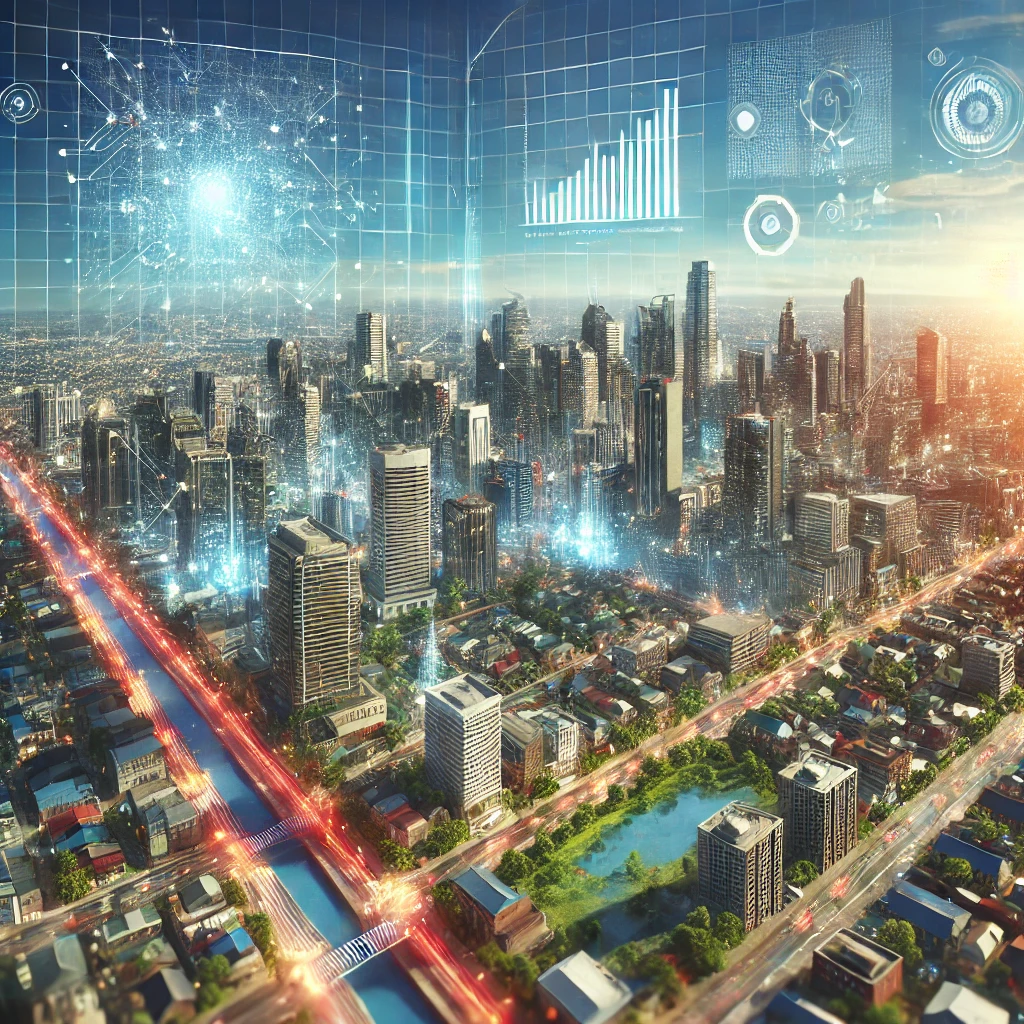
Satellite Imagery and Deep Learning for Smart City Insights
As cities grow at an unprecedented pace, the need for timely, data-driven insights has never been greater. Satellite imagery, combined with advanced deep learning techniques, is transforming how urban environments are understood and managed. From tracking land use and monitoring infrastructure to optimizing transportation and improving sustainability, these technologies empower cities to address challenges with precision and efficiency. With evolving machine learning methodologies and accessible AI-powered tools, such as customizable APIs, cities can now analyze vast amounts of data at scale. By embracing these innovations, urban planners and policymakers can build smarter, more inclusive and sustainable cities that are ready for the future.
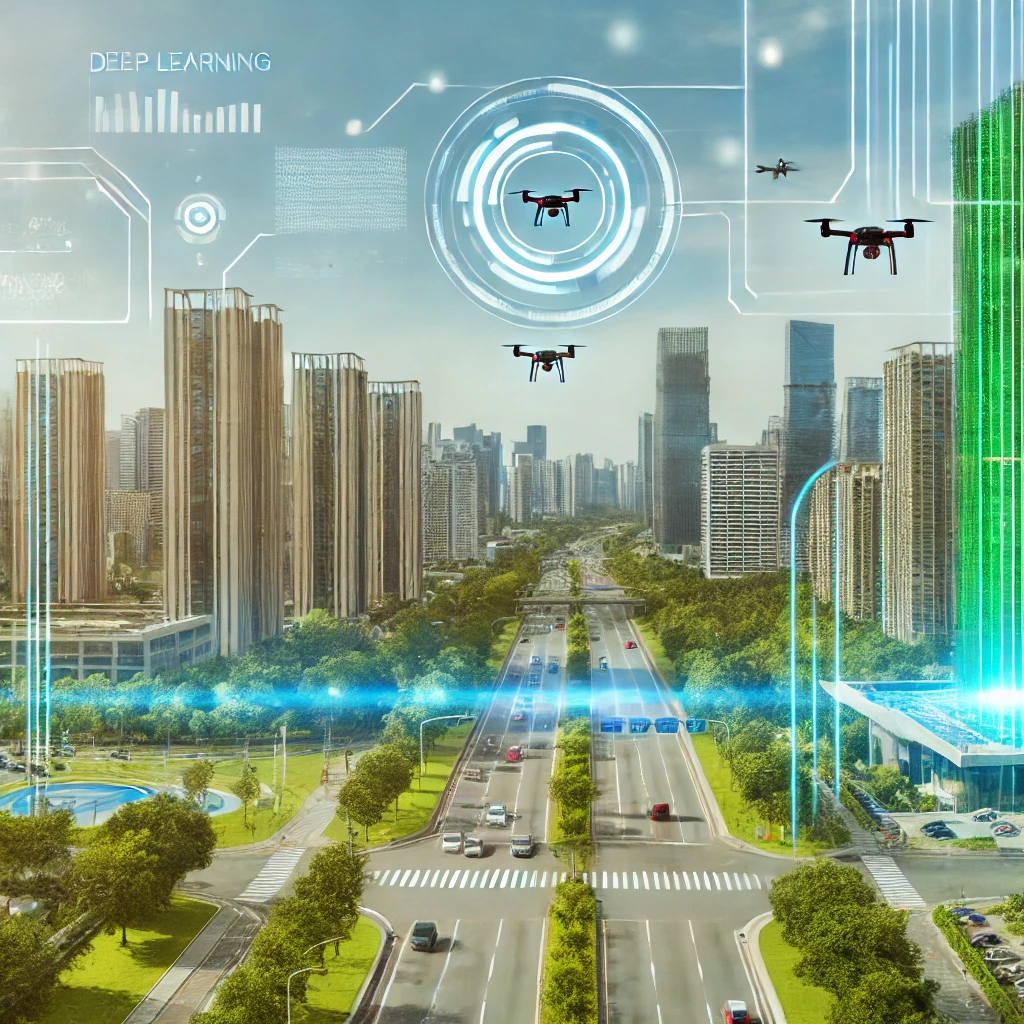
Deep Learning for Smart City Image Processing
Deep learning is transforming urban landscapes, powering smart city initiatives that improve efficiency, safety and sustainability. From real-time traffic management to advanced public safety systems, image processing technologies are enabling cities to make smarter, data-driven decisions. By combining ready-to-use AI APIs with tailored solutions, urban planners and developers can address unique challenges and scale innovations to meet growing demands. This blog explores how deep learning empowers smart cities, the challenges it faces, and the responsible deployment practices that ensure a brighter, more connected urban future.
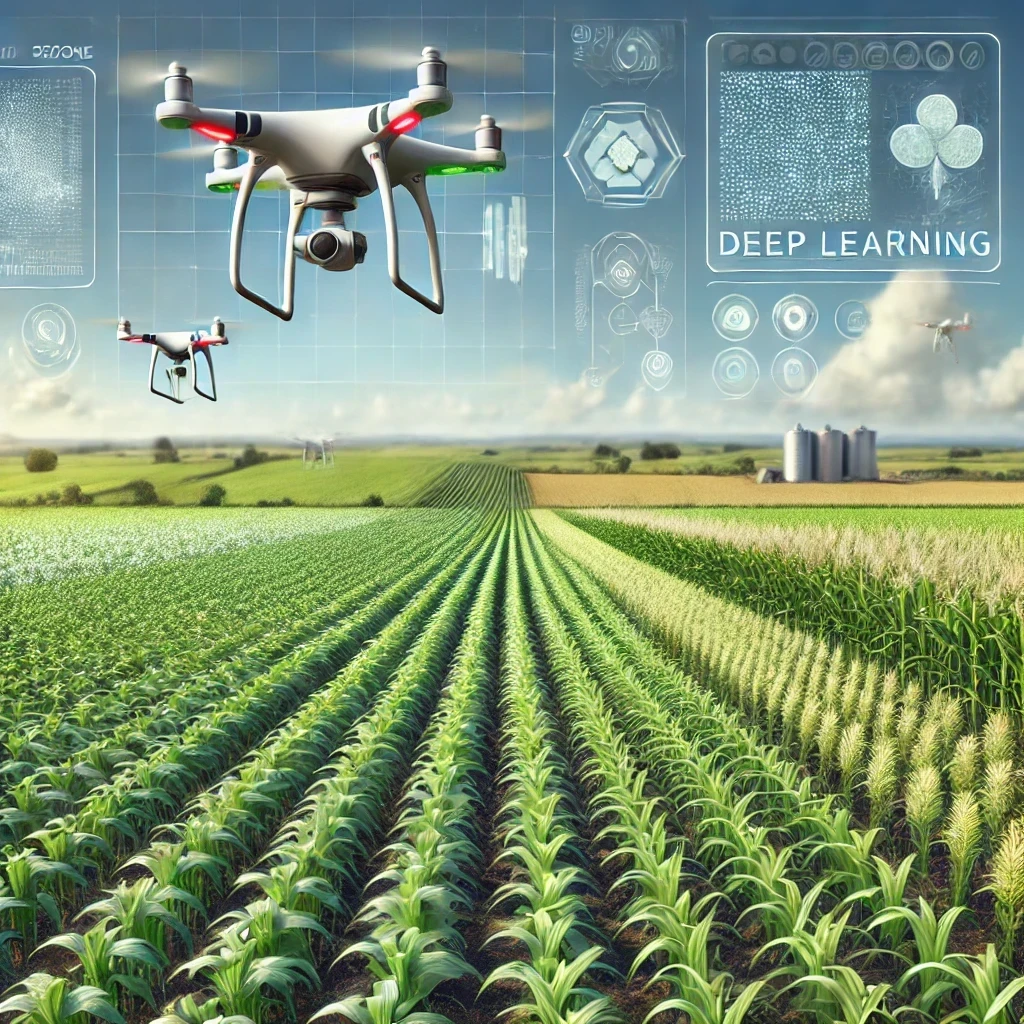
Enhancing Crop Monitoring with Deep Learning and Drone Imagery
AI-driven deep learning and drone imagery are transforming agriculture by addressing inefficiencies in traditional crop monitoring. These technologies empower farmers with real-time insights, enabling precise interventions to improve plant health, optimize resource usage and boost yields. With tailored AI solutions, businesses can overcome unique challenges and harness the full potential of smart agriculture. As innovation advances, the future of farming is poised for a sustainable and data-driven revolution.
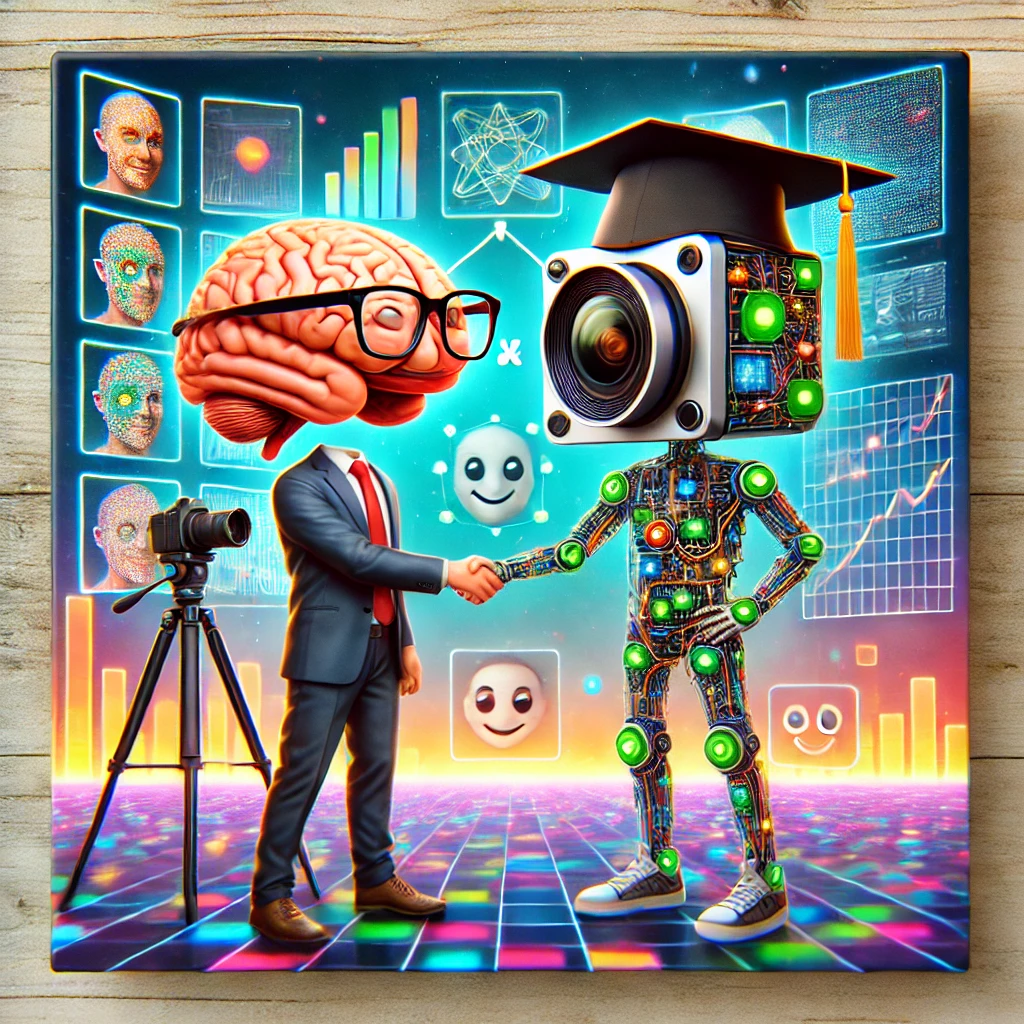
Computer Vision and Deep Learning: A Perfect Match
The synergy between computer vision and deep learning is transforming industries, enabling intelligent image analysis with unparalleled accuracy and scalability. From e-commerce and healthcare to transportation and security, discover how AI-driven solutions are solving real-world challenges, the latest innovations shaping the field and the benefits of tailored computer vision systems for unique business needs.